That led me down a rabbit hole. Here’s a hacked- together implementation of opt()
with minibatches, followed by a comparison on logistic regression. I have no idea what I’m doing when it comes to tuning stochastic optimisers, so I’m sure the comparison could be much better.
Shouldn’t be too hard to wrap this into the next release of greta, though I’d appreciate feedback on this approach before then!
opt <- function (model,
optimiser = bfgs(),
max_iterations = 100,
tolerance = 1e-06,
initial_values = initials(),
adjust = TRUE,
hessian = FALSE,
batch_fun = NULL) {
prep_initials <- greta:::prep_initials
# if there's a batch function, mess with the model, and later with the optimiser
if (!is.null(batch_fun)) {
if (optimiser$class$classname != "tf_optimiser") {
stop ("minibatching is only compatible with tensorflow optimisers, ",
"not scipy optimisers", call. = FALSE)
}
# find tensors corresponding to the minibatched data
example_batch <- batch_fun()
batched_greta_array_names <- names(example_batch)
batched_greta_arrays <- lapply(
batched_greta_array_names,
get,
envir = parent.frame()
)
tf_names <- vapply(
batched_greta_arrays,
function (ga) {
model$dag$tf_name(greta:::get_node(ga))
},
FUN.VALUE = character(1)
)
# replace the build_feed_dict function with a version that draws minibatches each time
build_feed_dict <- function (dict_list = list(),
data_list = self$tf_environment$data_list) {
tfe <- self$tf_environment
# replace appropriate elements of data_list with minibatches
batch <- batch_fun()
names(batch) <- tf_names
for (name in tf_names) {
data_list[[name]][] <- batch[[name]]
}
# put the list in the environment temporarily
tfe$dict_list <- c(dict_list, data_list)
on.exit(rm("dict_list", envir = tfe))
# roll into a dict in the tf environment
self$tf_run(feed_dict <- do.call(dict, dict_list))
}
# fix the environment of this function and put required information in there
dag_env <- model$dag$.__enclos_env__
environment(build_feed_dict) <- dag_env
dag_env$batch_fun <- batch_fun
dag_env$tf_names <- tf_names
# replace the functions with these new ones
unlockBinding("build_feed_dict", env = model$dag)
model$dag$build_feed_dict <- build_feed_dict
lockBinding("build_feed_dict", env = model$dag)
}
# check initial values. Can up the number of chains in the future to handle
# random restarts
initial_values_list <- prep_initials(initial_values, 1, model$dag)
# create R6 object of the right type
object <- optimiser$class$new(initial_values = initial_values_list[1],
model = model,
name = optimiser$name,
method = optimiser$method,
parameters = optimiser$parameters,
other_args = optimiser$other_args,
max_iterations = max_iterations,
tolerance = tolerance,
adjust = adjust)
# if there's a batch function, mess with the minimiser to change batch at
# every iteration
if (!is.null(batch_fun)) {
run_minimiser <- function() {
self$set_inits()
while (self$it < self$max_iterations &
self$diff > self$tolerance) {
self$it <- self$it + 1
self$model$dag$build_feed_dict()
self$model$dag$tf_sess_run(train)
if (self$adjust) {
obj <- self$model$dag$tf_sess_run(optimiser_objective_adj)
} else {
obj <- self$model$dag$tf_sess_run(optimiser_objective)
}
self$diff <- abs(self$old_obj - obj)
self$old_obj <- obj
}
}
environment(run_minimiser) <- object$.__enclos_env__
# replace the functions with these new ones
unlockBinding("run_minimiser", env = object)
object$run_minimiser <- run_minimiser
lockBinding("run_minimiser", env = object)
}
# run it and get the outputs
object$run()
outputs <- object$return_outputs()
# optionally evaluate the hessians at these parameters (return as hessian for
# objective function)
if (hessian) {
hessians <- model$dag$hessians()
hessians <- lapply(hessians, `*`, -1)
outputs$hessian <- hessians
}
outputs
}
# ~~~~~~~~~
# full dataset
set.seed(2019-06-29)
n <- 500000
k <- 50
X_big <- cbind(1, matrix(rnorm(n * k), n, k))
beta_true <- rnorm(k + 1, 0, 0.1)
y_big <- rbinom(n, 1, plogis(X_big %*% beta_true))
library(greta)
# ~~~~~~~~~
# model with all the data
beta <- normal(0, 10, dim = k + 1)
eta <- X_big %*% beta
p <- ilogit(eta)
distribution(y_big) <- bernoulli(p)
m_full <- model(beta)
# ~~~~~~~~~
# model with minibatches
# user-defined minibatching function (this could load minibatches from disk,
# rather than needing them to be defined in memory, or we could make use of TF's
# functionality for queuing batches)
get_minibatch <- function (size = 1000) {
idx <- sample.int(n, size)
list(X = X_big[idx, ], y = y_big[idx])
}
# use a single minibatch to set up the model with the correct dimensions. Must
# use as_data here so that the data can be swapped in, and the names of those
# greta arrays must match the names returned by get_minibatch
mini <- get_minibatch()
X <- as_data(mini$X)
y <- as_data(mini$y)
beta <- normal(0, 10, dim = k + 1)
eta <- X %*% beta
p <- ilogit(eta)
distribution(y) <- bernoulli(p)
m_mini <- model(beta)
# ~~~~~~~~~
# run the two optimisers and a glm
full_time <- system.time(
o_full <- opt(m_full)
)
mini_time <- system.time(
o_mini <- opt(
m_mini,
optimiser = adam(learning_rate = 0.01),
tolerance = 0,
max_iterations = 100,
batch_fun = get_minibatch
)
)
glm_time <- system.time(
o_glm <- glm(y_big ~ . - 1,
data = data.frame(X_big),
family = stats::binomial)
)
# visually compare them
plot(o_full$par$beta ~ beta_true)
points(o_glm$coefficients ~ beta_true, pch = 16, col = "blue", cex = 0.5)
points(o_mini$par$beta ~ beta_true, pch = 16, col = "red")
abline(0, 1)
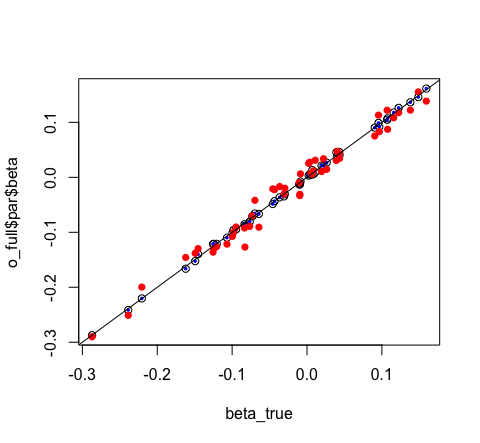
full_time
user system elapsed
74.351 13.486 41.860
mini_time
user system elapsed
2.178 0.203 1.581
glm_time
user system elapsed
7.757 1.116 8.890